
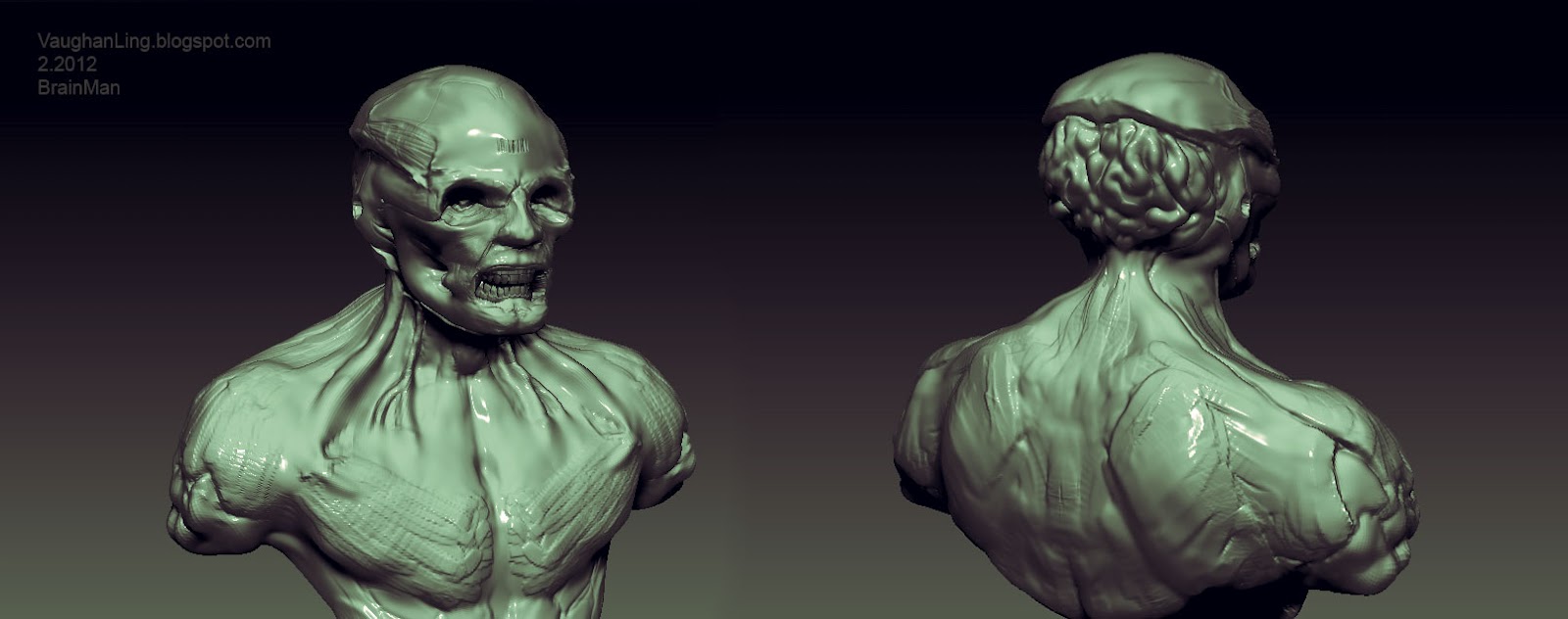
The ANN uses 24 past hourly solar wind parameters values to forecast the Dst index. Plain Language Summary We propose an artificial neural network for the prediction of disturbance storm time (Dst) index 1 to 12 hr ahead. In addition, the results show a strong dependence on the solar wind electric current. The results are outstanding in term of accuracy when considering a medium-term prediction of 12 hr in advance and in terms of timing of the Dst minimum occurrence. Generally, the duration and number of the input parameters significantly affect the training and prediction performance of the applied ANN. The power of the proposed ANN was illustrated using the strongest six storms recorded during the prediction period. The results indicate an adequate accuracy of R = 0.876 for prediction 2 hr in advance and R = 0.857 for prediction 12 hr in advance. Several ANN structures were tested and the best results were determined using the correlation coefficient (R) during the training and prediction phases. The ANN was trained on the data period was used to test the prediction capabilities of the ANN. The input parameters are the solar wind interplanetary magnetic field, north-south component of interplanetary magnetic field, temperature, density, speed, pressure, and electric field. The ANN uses past near-Earth solar wind parameter values to forecast the Dst. In this work, we propose an artificial neural network (ANN) with seven input parameters for the prediction of disturbance storm time (Dst) index 1 to 12 hr ahead. These neural networks can be applied on longer or shorter samples intervals of the solar wind data. The networks showed similar response, indicating the strong dependence of the Disturbance storm time index on the southward component of the interplanetary magnetic field, and the solar wind parameters (velocity, and pressure). Its precision was two hour later or ahead, which represents one sample difference between the observed and the predicted Dst indices. Also, it was detected the storm onset time with accurate timing. Moreover, it was predicted the minimum expected Dst value during the main phase with relatively accurate amplitude. Therefore, the ANN technique can provide real-time and accurate alerts even before the geomagnetic storm begins. The initial phase was predicted 3 h ahead. The applied ANNs showed a good performance in predicting the Dst index during the initial and main phases. In addition, we explained the performance of the neural networks during the extreme storm in each set. Thereafter, the neural networks were trained utilizing each data set. The study period was divided into four training short periods of 5 years and one predicted short period of 2 years. Meanwhile, we trained the second network using 3 parameters (the south component of interplanetary magnetic field, and 2 solar wind parameters (velocity, and pressure)). The first network had 7 input parameters, which are the interplanetary magnetic field, the south component of interplanetary magnetic field, and 5 solar wind parameters (temperature, density, velocity, pressure, and electric field). By using separate constitutes of the interplanetary data and the solar wind parameters, we tested two neural networks during the period from January 1996 to May 2017. The architecture of neural networks is time delay Feed-forward with one hidden layer. We designed two neural networks with the same structure and different number of input parameters. This study was carried out to achieve the prediction of the Disturbance storm time index variations based on one hour interplanetary data and solar wind parameters using artificial neural networks.
